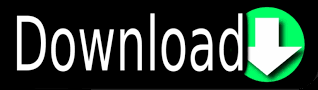
Spss logistic regression full#
The full content is now available from Statistical Associates Publishers.

For the same class of problems, logistic regression has become more popular among social scientists. Where both are applicable, logit regression has numerically equivalent results to logistic regression, but with different output options. Logit regression, discussed separately, is another related option in SPSS and other statistics packages for using loglinear methods to analyze one or more dependents. Goodness-of-fit tests such as the likelihood ratio test are available as indicators of model appropriateness, as is the Wald statistic to test the significance of individual independent variables. The predictive success of the logistic regression can be assessed by looking at the classification table, showing correct and incorrect classifications of the dichotomous, ordinal, or polytomous dependent. It does, however, require that observations be independent and that the independent variables be linearly related to the logit of the dependent. Unlike OLS regression, however, logistic regression does not assume linearity of relationship between the raw values of the independent variables and raw values of the dependent, does not require normally distributed variables, does not assume homoscedasticity, and in general has less stringent requirements. Logistic regression has many analogies to OLS regression: logit coefficients correspond to b coefficients in the logistic regression equation, the standardized logit coefficients correspond to beta weights, and a pseudo R2 statistic is available to summarize the strength of the relationship. This means that logistic regression calculates changes in the log odds of the dependent, not changes in the dependent itself as OLS regression does. Logistic regression estimates the odds of a certain event (value) occurring. A logit is the natural log of the odds of the dependent equaling a certain value or not (usually 1 in binary logistic models, or the highest value in multinomial models). Logistic regression applies maximum likelihood estimation after transforming the dependent into a logit variable.

The impact of predictor variables is usually explained in terms of odds ratios. Logistic regression can be used to predict a categorical dependent variable on the basis of continuous and/or categorical independents to determine the effect size of the independent variables on the dependent to rank the relative importance of independents to assess interaction effects and to understand the impact of covariate control variables. Similarly, generalized linear mixed modeling (GLMM) is now available to handle multilevel logistic modeling.
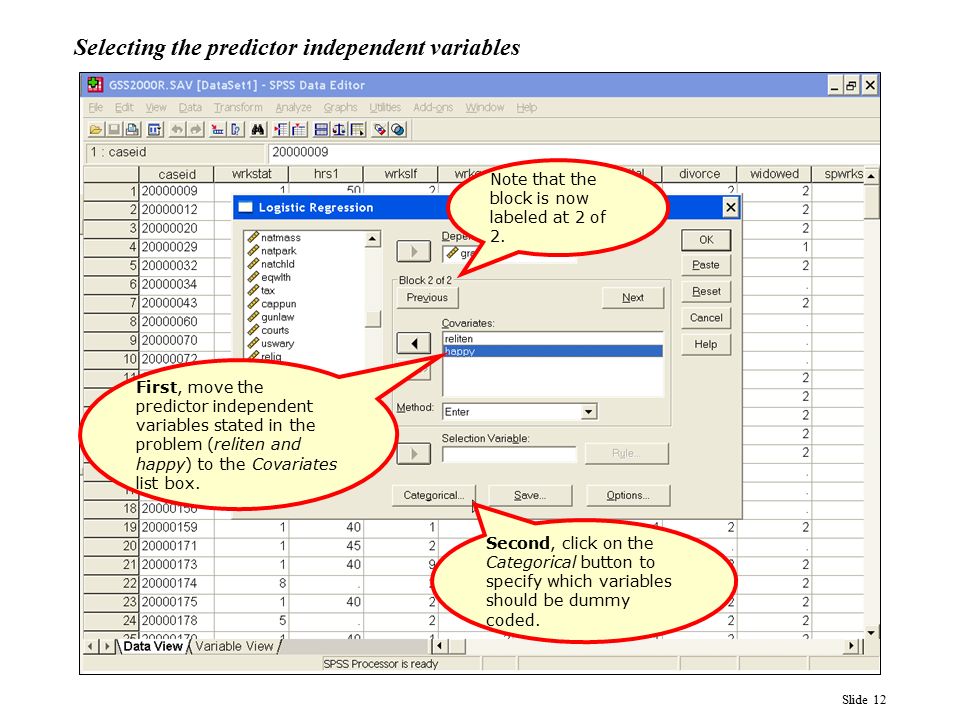
GZLM provides allows the researcher to create regression models with any distribution of the dependent (ex., binary, multinomial, ordinal) and any link function (ex., log for loglinear analysis, logit for binary or multinomial logistic analysis, cumulative logit for ordinal logistic analysis). More recently, generalized linear modeling (GZLM) has appeared as a module in SPSS, SAS, and other packages. Unlike logit regression, there can be only one dependent variable. Note that continuous variables are not used as dependents in logistic regression. When multiple classes of a multinomial dependent variable can be ranked, then ordinal logistic regression is preferred to multinomial logistic regression since ordinal regression has higher power for ordinal data. Multinomial logistic regression exists to handle the case of dependents with more classes than two, though it is sometimes used for binary dependents also since it generates somewhat different output described below. Binary logistic regression is a form of regression which is used when the dependent is a dichotomy and the independents are of any type.
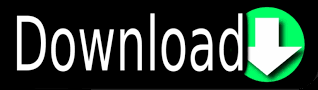